第五十六讲——威斯康星大学麦迪逊分校张正军教授、中山大学王学钦教授和悉尼大学王启应教授
2019年6月20日上午,应4688美高梅集团唯一网站和岭南统计科学研究中心的邀请,在行政东412会议室,威斯康星大学麦迪逊分校张正军教授、中山大学王学钦教授和悉尼大学王启应教授分别作了“Mark to Market Value at Risk”、“Hierarchical Change-Point Detection for Multivariate Time Series via a Ball Detection Function”和“Current developments in nonlinear cointegrating regression”的学术报告——暨“羊城讲坛”第五十六讲,旨在进一步提高年轻学者及研究生对相关研究的理解。此次讲座由孙六全教授主持,相关专业的师生参加了此次讲座。

张正军,统计学博士,美国威斯康星大学麦迪逊分校统计系教授。研究领域包括金融时间序列分析、极值理论、金融风险分析、贝叶斯统计等。目前担任美国威斯康星大学统计系副主任、招生委员会主任、国际商务及经济期刊Journal of Business & Economic Statistics、国际概率统计期刊JKSS、Statistics and Its Interface等多个国际SCI期刊副主编。

报告主要内容:Financial risk management has been overwhelmed by applications and research of value at risk (VaR) in daily practice mainly due to its simple form and easily interpretable feature. Yet, its serious drawback of underestimating an asset's market risk has been noticed in numerous applications, and many alternative risk measures have been proposed in the literature. Among all existing alternative risk measures, it is hard to find one that a financial institution whose portfolio has multiple settlements before the end of holding period uses to internally perform risk assessment. We propose a new risk measure termed mark to market value at risk (MMVaR) for settlement being taken daily during the holding period. MMVaR is a natural alternative risk measure to VaR as it is a direct generalization of VaR. It not only maintains easily interpretable feature held by VaR, but also better computes an asset's market risk in a financial institution having daily account settlements. We show that MMVaR is superior to VaR using simulation examples and real data. In real data analysis, we find that risks calculated usingMMVaR are about 20% higher than risks calculated using classical VaR, wMMVaR are about 20% higher than risks calculated using classical VaR, which provides an evidence proof of Basel III's new capital adequacy ratio requirement, and hence it can become an implementable daily risk measure.

王学钦教授,中山大学数学学院和中山医学院双聘教授,博士生导师;中山大学统计学科带头人;中山大学华南统计科学研究中心执行主任;国家优秀青年基金获得者,教育部新世纪人才,教育部统计专业教指委委员;研究领域为非参多元统计学、统计学习、和精准医学。
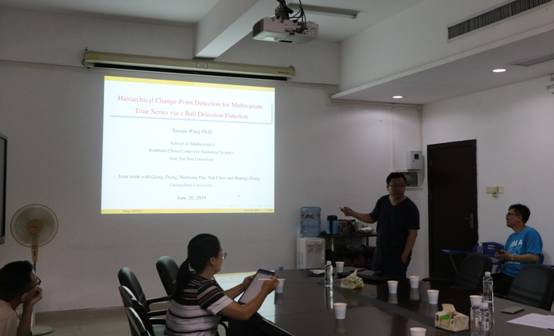
报告主要内容:Sequences of random objects arise from many real applications, including high throughput omic data and functional imaging data. Those sequences are usually dependent, non-linear, or even Non-Euclidean, and an important problem is change-point detection in such dependent sequences in Banach spaces or metric spaces. The problem usually requires the accurate inference for not only whether changes might have occurred but also the locations of the changes when they did occur. To this end, we first introduce a Ball detection function and show that it reaches its maximum at the change-point if a sequence has only one change point. Furthermore, we propose a consistent estimator of Ball detection function based on which we develop a hierarchical algorithm to detect all possible change points. We prove that the estimated change-point locations are consistent. Our procedure can estimate the number of change-points and detect their locations without assuming any particular types of change-points as a change can occur in a sequence in different ways. Extensive simulation studies and analyses of two interesting real datasets wind direction and Bitcoin price demonstrate that our method has considerable advantages over existing competitors, especially when data are non-Euclidean or when there are distributional changes in the variance.

王启应,2001年获得澳大利亚University of Wollongong大学博士学位,随后在澳大利亚悉尼大学数学与统计学院工作。主要研究方向为:Nonstationary time series econometrics, Financial Econometrics, Nonparametric statistics, Econometric Theory, Self-normalized limit theory.王启应教授在概率统计学与计量经济学顶级专业学术期刊The Annals of Probability, The Annals of Statistics、Econometric Theory以及 Journal of Econometrics 等杂志上发表论文数十篇,成果显著。

报告主要内容:The past decade has witnessed great progress in the development of nonlinear cointegrating regression. Unlike linear cointegration and nonlinear regression with stationarity where the traditional and classical methods are widely used in practice, estimation and inference theory in nonlinear cointegrating regression produce new mechanisms involving local time, a mixture of normal distributions and stochastic integrals. This talk aims to introduce the machinery of the theoretical developments, providing up-to-date results in nonlinear cointegrating regression.